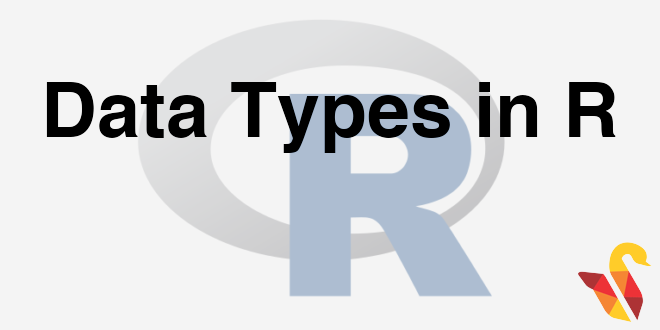
In previous section, we studied about R Packages, now we will be studying about R Data Types.
This is the most important topic of our current session. R has vectors, data frames, lists.
R Vectors
Everything in R is stored as a Vector by default, most of the times.
If I say my name:
>name<-“karthik”
The structure of name, Str(name) is Char
The data-type of name, is.vector(name)-TRUE. So, by default everything we create is a vector.
>Age<-29 >Is.vector(age):TRUE
Thus everything is a vector; basically, a vector is a combination/group of all basic elements that are put together. There are many advantages of using Vector like we need not write loops for making operations on vectors. c() is a concatenation operator. We can also say that vectors are a generalized version for arrays.
>Age <- c(15, 17, 16, 15, 16) >English<- c(40, 56, 30, 68, 35) >Science<- c(85, 80, 74, 39, 65) >Name<- c("John", "Bob", "Kevin", "Smith", "Rick") >is.vector(Age) >True >is.vector(English) >True >is.vector(Name) >True
Many mathematical functions can be applied without loops. If we want to add 3 to vector-age, we need not use loop and run, simply we can write :
>Age+3 [1] 18 20 19 18 19 >English1<- English+10 >Total<- English1 + Science >Total [1] 135 146 114 117 110 >Age/Total [1] 0.1111111 0.1164384 0.1403509 0.1282051 0.1454545
Another example is as below
>x <- rnorm(100,mean=20,sd=5) >mean(x) [1] 20.16493 >x-mean(x)
## [1] -0.56962602 1.20292587 -7.99204832 2.82564771 1.18117481 ## [6] -5.24518066 -2.68735732 -3.27495626 -0.28558601 0.74857039 ## [11] -0.47749603 -10.15470947 -1.06874992 2.31996526 2.84350465 ## [16] 8.16148504 0.25051846 6.19313835 2.59168828 0.29868066 ## [21] -3.82597413 5.39263180 4.67593290 -0.43454849 -8.25625452 ## [26] 7.84636863 -1.06371741 0.50440888 -3.09572178 3.99628700 ## [31] 9.38662504 -2.09353644 -1.95195873 8.14326027 -6.18426536 ## [36] 3.46082316 2.48187522 -11.74572064 0.35223491 1.67869236 ## [41] -6.35170720 -1.45205184 2.91846245 -1.49338586 1.11208055 ## [46] -8.72848627 -7.52563928 6.15454046 -1.22060564 10.02838035 ## [51] 1.70506238 1.41094805 -10.72651241 -0.66319353 -7.20567753 ## [56] 2.73986617 2.68289335 -2.83861944 -8.99196764 3.97441791 ## [61] -0.74130073 8.79819626 -14.87133600 3.95593514 1.10624785 ## [66] -2.89865994 -3.68172179 0.41355051 9.62255710 -9.98099032 ## [71] 12.66459589 4.30046314 9.53252294 -3.40578675 -1.04795097 ## [76] -3.09459639 -0.24209619 4.98138610 -0.70323448 -4.26956279 ## [81] 3.35833387 5.09742073 -14.16394754 4.97869260 1.15177611 ## [86] 0.60028795 -4.74663476 -1.96627009 5.71759434 0.02098097 ## [91] -0.49594059 -3.92591152 -6.29043650 -5.41646894 -2.31449988 ## [96] 1.87594179 8.00307231 3.92296652 10.13654537 2.36044150
Accessing of vector elements
We need to use the [] operator to access the elements
>Age [1] 15 17 16 15 16
For accessing the third element of Age vector
>Age[3] [1] 16
The 2nd, 3rd, 4th and 5th elements of the Age vector
Age[2:5] [1] 17 16 15 16
The 1st, 3rd and 5th elements of Age vector
>Age[c(1,3,5)] [1] 15 16 16
To eliminate or ignore the second value and get rest of values.
>Age[-2] [1] 15 16 15 16
Replace 3rd element with 19
>Age[3]<-19
>Age
[1] 15 17 19 15 16
Adding a new element to the vector
>Age[6]<-22 >Age [1] 15 17 19 15 16 22
To introduce value to an arbitrary position ,
>Age[10]<-42 >Age [1] 15 17 19 15 16 22 NA NA NA 42
Every other value will be NA and 10th element will be 42.
We can also try to give all these numbers in cluster
>Age[6:10]<-c(23,25,26,29,33,35) >Age [1] 15 17 19 15 16 23 25 26 29 33 35
To find the vector type, We use the Class() of the vector to find the vector type
>class(Age) [1]"numeric" >class(Name) [1]"character"
The succeeding posts will discuss on Data Frames.
In next section, we will be studying about R Data Frames.