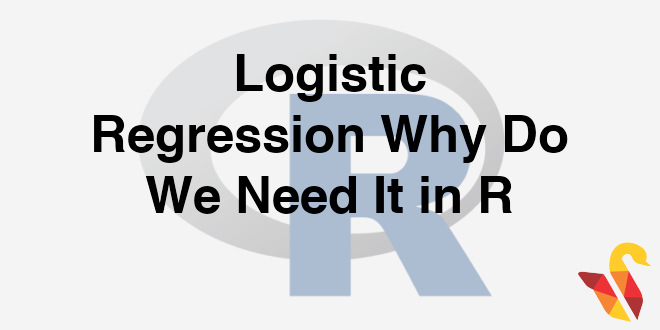
Regression Recap
In previous section, we studied about Linear Regression with Multicollinearity in R and Conclusion
- Dependent variable is predicted using independent variables
- A straight line is fit to capture the relation in the form of a model
- The R-Square/ Adjusted R-Square values tell us the goodness of fit of the model
- Once the line is ready we can substitute the values of x(predictor) to get the predicted values of y(dependent variable)
LAB: Regression – Recap
-
- Import Dataset: Product Sales Data/Product_sales.csv
-
- What are the variables in the dataset?
-
- Build a predictive model for Bought vs Age
-
- What is R-Square?
-
- If Age is 4 then will that customer buy the product?
-
- If Age is 105 then will that customer buy the product?
-
- Draw a scatter plot between Age and Buy. Include the regression line on the same chart.
Solution
-
- Import Dataset: Product Sales Data/Product_sales.csv
Product_sales <- read.csv("C:\\Amrita\\Datavedi\\Product Sales Data\\Product_sales.csv")
-
- What are the variables in the dataset?
names(Product_sales)
## [1] "Age" "Bought"
-
- Build a predictive model for Bought vs Age
prod_sales_model<-lm(Bought~Age,data=Product_sales)
summary(prod_sales_model)
##
## Call:
## lm(formula = Bought ~ Age, data = Product_sales)
##
## Residuals:
## Min 1Q Median 3Q Max
## -1.14894 -0.12800 -0.01807 0.10759 1.10759
##
## Coefficients:
## Estimate Std. Error t value Pr(>|t|)
## (Intercept) -0.1704125 0.0152752 -11.16 <2e-16 ***
## Age 0.0209421 0.0004205 49.80 <2e-16 ***
## ---
## Signif. codes: 0 '***' 0.001 '**' 0.01 '*' 0.05 '.' 0.1 ' ' 1
##
## Residual standard error: 0.1976 on 465 degrees of freedom
## Multiple R-squared: 0.8421, Adjusted R-squared: 0.8418
## F-statistic: 2480 on 1 and 465 DF, p-value: < 2.2e-16
-
- What is R-Square?
0.8421 – 5. If Age is 4 then will that customer buy the product?
new_data<-data.frame(Age=4)
predict(prod_sales_model,new_data)
## 1
## -0.08664394
-
- If Age is 105 then will that customer buy the product?
new_data<-data.frame(Age=105)
predict(prod_sales_model,new_data)
## 1
## 2.028511
-
- Draw a scatter plot between Age and Buy. Include the regression line on the same chart.
plot(Product_sales$Age,Product_sales$Bought,col = "blue")
abline(prod_sales_model, lwd = 5, col="red")
What is the need of logistic regression?
- Consider Product sales data. The dataset has two columns.
- Age – continuous variable between 6-80
- Buy(0- Yes ; 1-No)
plot(Product_sales$Age,Product_sales$Bought,col = "blue")
Real-life examples
- Gaming – Win vs. Loss
- Sales – Buying vs. Not buying
- Marketing – Response vs. No Response
- Credit card & Loans – Default vs. Non Default
- Operations – Attrition vs. Retention
- Websites – Click vs. No click
- Fraud identification – Fraud vs. Non Fraud
- Healthcare – Cure vs. No Cure
Why not linear?
Some Nonlinear Functions
A Logistic Function
The Logistic Function
- We want a model that predicts probabilities between 0 and 1, that is, S-shaped.
- There are lots of s-shaped curves. We use the logistic model:
- \[Probability = \frac{e^{(\beta_0+ \beta_1X)}}{1+e^{($\beta_0+ \beta_1X)}}\]
- \[log_e(\frac{P}{1-P})=\beta_0+\beta_1X\]
- The function on left, \(log_e(\frac{P}{1-P})\), is called the logistic function.
The next post is about logistic function to regression.