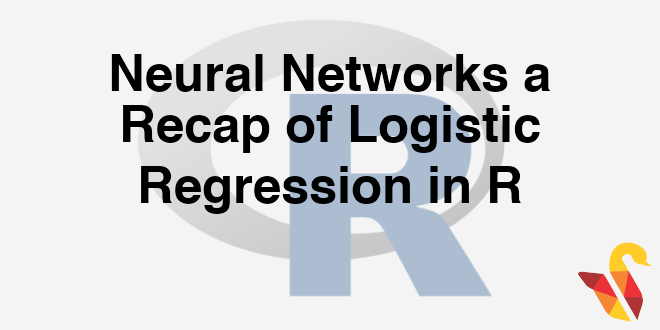
In previous section, we studied about Cross Validation
In this post we will just revise our understanding of how logistic regression works, which can be considered a building block for a neural network.
Contents
- Neural network Intuition
- Neural network and vocabulary
- Neural network algorithm
- Math behind neural network algorithm
- Building the neural networks
- Validating the neural network model
- Neural network applications
- Image recognition using neural networks
Recap of Logistic Regression
- Categorical output YES/NO type
- Using the predictor variables to predict the categorical output
LAB: Logistic Regression
- Dataset: Emp_Productivity/Emp_Productivity.csv
- Filter the data and take a subset from above dataset . Filter condition is Sample_Set<3
- Draw a scatter plot that shows Age on X axis and Experience on Y-axis. Try to distinguish the two classes with colors or shapes (visualizing the classes)
- Build a logistic regression model to predict Productivity using age and experience
- Finally draw the decision boundary for this logistic regression model
- Create the confusion matrix
- Calculate the accuracy and error rates
Solution
Emp_Productivity_raw <- read.csv("C:\\Amrita\\Datavedi\\Emp_Productivity\\Emp_Productivity.csv")
- Filter the data and take a subset from above dataset . Filter condition is Sample_Set<3
Emp_Productivity1<-Emp_Productivity_raw[Emp_Productivity_raw$Sample_Set<3,]
dim(Emp_Productivity1)
## [1] 74 4
names(Emp_Productivity1)
## [1] "Age" "Experience" "Productivity" "Sample_Set"
head(Emp_Productivity1)
## Age Experience Productivity Sample_Set
## 1 20.0 2.3 0 1
## 2 16.2 2.2 0 1
## 3 20.2 1.8 0 1
## 4 18.8 1.4 0 1
## 5 18.9 3.2 0 1
## 6 16.7 3.9 0 1
table(Emp_Productivity1$Productivity)
##
## 0 1
## 33 41
- Draw a scatter plot that shows Age on X axis and Experience on Y-axis. Try to distinguish the two classes with colors or shapes (visualizing the classes)
library(ggplot2)
ggplot(Emp_Productivity1)+geom_point(aes(x=Age,y=Experience,color=factor(Productivity),shape=factor(Productivity)),size=5)
– Build a logistic regression model to predict Productivity using age and experience
Emp_Productivity_logit<-glm(Productivity~Age+Experience,data=Emp_Productivity1, family=binomial())
Emp_Productivity_logit
##
## Call: glm(formula = Productivity ~ Age + Experience, family = binomial(),
## data = Emp_Productivity1)
##
## Coefficients:
## (Intercept) Age Experience
## -8.9361 0.2763 0.5923
##
## Degrees of Freedom: 73 Total (i.e. Null); 71 Residual
## Null Deviance: 101.7
## Residual Deviance: 46.77 AIC: 52.77
coef(Emp_Productivity_logit)
## (Intercept) Age Experience
## -8.9361114 0.2762749 0.5923444
slope1 <- coef(Emp_Productivity_logit)[2]/(-coef(Emp_Productivity_logit)[3])
intercept1 <- coef(Emp_Productivity_logit)[1]/(-coef(Emp_Productivity_logit)[3])
- Finally draw the decision boundary for this logistic regression model
library(ggplot2)
base<-ggplot(Emp_Productivity1)+geom_point(aes(x=Age,y=Experience,color=factor(Productivity),shape=factor(Productivity)),size=5)
base+geom_abline(intercept = intercept1 , slope = slope1, color = "red", size = 2) #Base is the scatter plot. Then we are adding the decision boundary
– Create the confusion matrix
predicted_values<-round(predict(Emp_Productivity_logit,type="response"),0)
conf_matrix<-table(predicted_values,Emp_Productivity_logit$y)
conf_matrix
##
## predicted_values 0 1
## 0 31 2
## 1 2 39
- Calculate the accuracy and error rates
accuracy<-(conf_matrix[1,1]+conf_matrix[2,2])/(sum(conf_matrix))
accuracy
## [1] 0.9459459
- The next post is about Decision Boundary Logistic Regression.