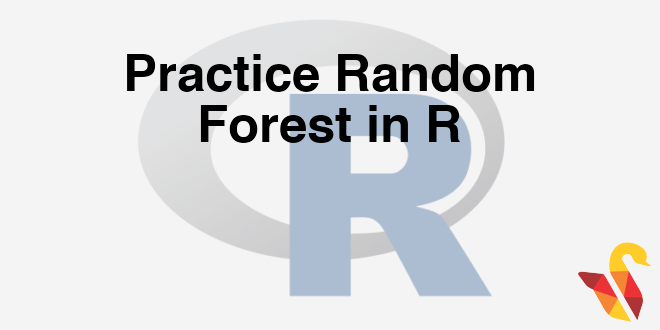
In previous section, we studied about The Random Forest
Let’s implement the concept of Random Forest into practice using R.
LAB: Random Forest
- Dataset: /Car Accidents IOT/Train.csv
- Build a decision tree model to predict the fatality of accident
- Build a decision tree model on the training data.
- On the test data, calculate the classification error and accuracy.
- Build a random forest model on the training data.
- On the test data, calculate the classification error and accuracy.
- What is the improvement of the Random Forest model when compared with the single tree?
Solution
#Data Import
train<- read.csv("C:/Amrita/Datavedi/Car Accidents IOT/Train.csv")
test<- read.csv("C:/Amrita/Datavedi/Car Accidents IOT/Test.csv")
dim(train)
## [1] 15109 23
head(train)
## Fatal S1 S2 S3 S4 S5 S6 S7 S8 S9 S10 S11
## 1 1 36.2247 10.77330 0.243897 596 100.6710 0 0 1 28 0.016064 313
## 2 1 35.7343 17.45510 0.243897 600 100.0000 0 0 1 14 0.015812 319
## 3 1 31.6561 7.61366 0.308763 604 99.3377 0 0 1 4 0.015560 323
## 4 1 33.8320 13.11190 0.293195 616 97.4026 0 0 1 8 0.016001 320
## 5 1 42.5138 13.99850 0.259465 632 94.9367 0 0 1 8 0.016064 322
## 6 1 36.1261 14.85930 0.278925 600 100.0000 0 0 1 4 0.015749 314
## S12 S13 S14 S15 S16 S17 S18 S19 S20 S21 S22
## 1 1 1 57 0 0.280 240 5.99375 0 0.0 4 14.9382
## 2 1 1 57 0 0.175 240 5.99375 0 0.0 4 14.8827
## 3 1 1 58 0 0.280 240 5.99375 0 0.0 4 14.6005
## 4 1 1 58 0 0.385 240 4.50625 0 13.0 4 14.6782
## 5 1 1 57 0 0.070 240 5.99375 0 19.5 4 15.3461
## 6 1 1 58 0 0.175 1008 4.50625 0 23.9 4 15.0559
###Decision Tree
library(rpart)
crash_model_ds<-rpart(Fatal ~ ., method="class", control=rpart.control(minsplit=30, cp=0.03), data=train)
#Training accuarcy
predicted_y<-predict(crash_model_ds, type="class")
table(predicted_y)
## predicted_y
## 0 1
## 5745 9364
confusionMatrix(predicted_y,train$Fatal)
## Confusion Matrix and Statistics
##
## Reference
## Prediction 0 1
## 0 4735 1010
## 1 1581 7783
##
## Accuracy : 0.8285
## 95% CI : (0.8224, 0.8345)
## No Information Rate : 0.582
## P-Value [Acc > NIR] : < 2.2e-16
##
## Kappa : 0.643
## Mcnemar's Test P-Value : < 2.2e-16
##
## Sensitivity : 0.7497
## Specificity : 0.8851
## Pos Pred Value : 0.8242
## Neg Pred Value : 0.8312
## Prevalence : 0.4180
## Detection Rate : 0.3134
## Detection Prevalence : 0.3802
## Balanced Accuracy : 0.8174
##
## 'Positive' Class : 0
##
#Accuaracy on Test data
predicted_test_ds<-predict(crash_model_ds, test, type="class")
confusionMatrix(predicted_test_ds,test$Fatal)
## Confusion Matrix and Statistics
##
## Reference
## Prediction 0 1
## 0 2897 561
## 1 995 4612
##
## Accuracy : 0.8284
## 95% CI : (0.8204, 0.8361)
## No Information Rate : 0.5707
## P-Value [Acc > NIR] : < 2.2e-16
##
## Kappa : 0.6448
## Mcnemar's Test P-Value : < 2.2e-16
##
## Sensitivity : 0.7443
## Specificity : 0.8916
## Pos Pred Value : 0.8378
## Neg Pred Value : 0.8225
## Prevalence : 0.4293
## Detection Rate : 0.3196
## Detection Prevalence : 0.3815
## Balanced Accuracy : 0.8179
##
## 'Positive' Class : 0
##
###Random Forest
library(randomForest)
## randomForest 4.6-12
## Type rfNews() to see new features/changes/bug fixes.
##
## Attaching package: 'randomForest'
## The following object is masked from 'package:ggplot2':
##
## margin
rf_model <- randomForest(as.factor(train$Fatal) ~ ., ntree=200, mtry=ncol(train)/3, data=train)
#Training accuaracy
predicted_y<-predict(rf_model)
table(predicted_y)
## predicted_y
## 0 1
## 5921 9188
confusionMatrix(predicted_y,train$Fatal)
## Confusion Matrix and Statistics
##
## Reference
## Prediction 0 1
## 0 5600 321
## 1 716 8472
##
## Accuracy : 0.9314
## 95% CI : (0.9272, 0.9353)
## No Information Rate : 0.582
## P-Value [Acc > NIR] : < 2.2e-16
##
## Kappa : 0.8577
## Mcnemar's Test P-Value : < 2.2e-16
##
## Sensitivity : 0.8866
## Specificity : 0.9635
## Pos Pred Value : 0.9458
## Neg Pred Value : 0.9221
## Prevalence : 0.4180
## Detection Rate : 0.3706
## Detection Prevalence : 0.3919
## Balanced Accuracy : 0.9251
##
## 'Positive' Class : 0
##
#Accuaracy on Test data
predicted_test_rf<-predict(rf_model,test, type="class")
confusionMatrix(predicted_test_rf,test$Fatal)
## Confusion Matrix and Statistics
##
## Reference
## Prediction 0 1
## 0 3479 192
## 1 413 4981
##
## Accuracy : 0.9333
## 95% CI : (0.9279, 0.9383)
## No Information Rate : 0.5707
## P-Value [Acc > NIR] : < 2.2e-16
##
## Kappa : 0.8628
## Mcnemar's Test P-Value : < 2.2e-16
##
## Sensitivity : 0.8939
## Specificity : 0.9629
## Pos Pred Value : 0.9477
## Neg Pred Value : 0.9234
## Prevalence : 0.4293
## Detection Rate : 0.3838
## Detection Prevalence : 0.4050
## Balanced Accuracy : 0.9284
##
## 'Positive' Class : 0
##
We can see an improvement in the Accuracy
The next post is on Boosting.