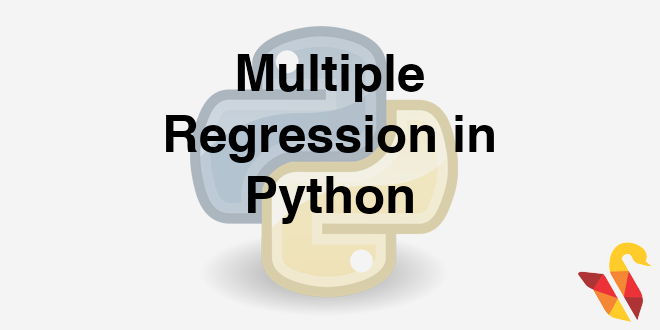
Link to the previous post: https://statinfer.com/204-1-5-r-squared-in-python/
For the last few posts of the machine learning blog series 204, we were just going through single input variable regression. In this post, we will see how to take care of multiple input variables.
Multiple Regression
- Using multiple predictor variables instead of single variable
- We need to find a perfect plane here
Code – Multiple Regression
In [21]:
from sklearn.linear_model import LinearRegression
lr = LinearRegression()
lr.fit(air[["Promotion_Budget"]+["Inter_metro_flight_ratio"]], air[["Passengers"]])
Out[21]:
In [22]:
predictions = lr.predict(air[["Promotion_Budget"]+["Inter_metro_flight_ratio"]])
In [23]:
import statsmodels.formula.api as sm
model = sm.ols(formula='Passengers ~ Promotion_Budget+Inter_metro_flight_ratio', data=air)
fitted = model.fit()
fitted.summary()
Out[23]:
Individual Impact of variables
- Look at the P-value
- Probability of the hypothesis being right.
- Individual variable coefficient is tested for significance
- Beta coefficients follow t distribution.
- Individual P values tell us about the significance of each variable
- A variable is significant if P value is less than 5%. Lesser the P-value, better the variable
- Note it is possible all the variables in a regression to produce a great fit, and yet very few of the variables be individually significant.
To test
H0:βi=0
Ha:βi≠0
Test Statistic
t=bi/s(bi)
Reject H0 if
t>t(α2;n−k−1)
or
t>−t(α2;n−k−1)
Practice : Multiple Regression
- Build a multiple regression model to predict the number of passengers
- What is R-square value
- Are there any predictor variables that are not impacting the dependent variable
In [24]:
#Build a multiple regression model to predict the number of passengers
from sklearn.linear_model import LinearRegression
lr = LinearRegression()
lr.fit(air[["Promotion_Budget"]+["Inter_metro_flight_ratio"]+["Service_Quality_Score"]], air[["Passengers"]])
predictions = lr.predict(air[["Promotion_Budget"]+["Inter_metro_flight_ratio"]+["Service_Quality_Score"]])
In [25]:
import statsmodels.formula.api as sm
model = sm.ols(formula='Passengers ~ Promotion_Budget+Inter_metro_flight_ratio+Service_Quality_Score', data=air)
fitted = model.fit()
fitted.summary()
Out[25]:
- What is R-square value
0.951
- Are there any predictor variables that are not impacting the dependent variable
Inter_metro_flight_ratio
The next post is about adjusted R squared in python.
Link to the next post: https://statinfer.com/204-1-7-adjusted-r-squared-in-python/