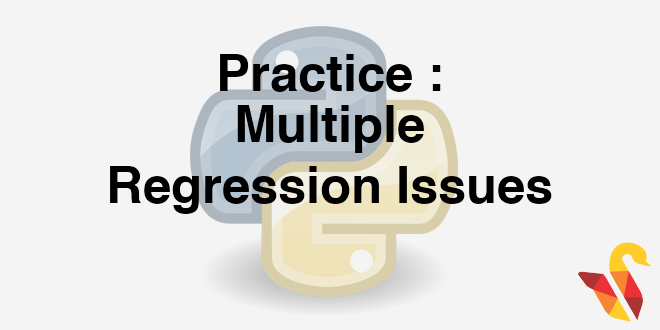
Link to the previous post: https://statinfer.com/204-1-7-adjusted-r-squared-in-python/
In the last post of this session, we did cover basics of Multiple variable Linear Regression. In this post, we will Practice and try to solve issues associated with Multiple Regression.
Practice : Multiple Regression- issues
- Import Final Exam Score data
- Build a model to predict final score using the rest of the variables.
- How are Sem2_Math & Final score related? As Sem2_Math score increases, what happens to Final score?
- Remove “Sem1_Math” variable from the model and rebuild the model
- Is there any change in R square or Adj R square
- How are Sem2_Math & Final score related now? As Sem2_Math score increases, what happens to Final score?
- Draw a scatter plot between Sem1_Math & Sem2_Math
- Find the correlation between Sem1_Math & Sem2_Math
In [34]:
#Import Final Exam Score data
final_exam=pd.read_csv("datasets\\Final Exam\\Final Exam Score.csv")
In [35]:
#Size of the data
final_exam.shape
Out[35]:
In [36]:
#Variable names
final_exam.columns
Out[36]:
In [37]:
#Build a model to predict final score using the rest of the variables.
from sklearn.linear_model import LinearRegression
lr1 = LinearRegression()
lr1.fit(final_exam[["Sem1_Science"]+["Sem2_Science"]+["Sem1_Math"]+["Sem2_Math"]], final_exam[["Final_exam_marks"]])
predictions1 = lr1.predict(final_exam[["Sem1_Science"]+["Sem2_Science"]+["Sem1_Math"]+["Sem2_Math"]])
import statsmodels.formula.api as sm
model1 = sm.ols(formula='Final_exam_marks ~ Sem1_Science+Sem2_Science+Sem1_Math+Sem2_Math', data=final_exam)
fitted1 = model1.fit()
fitted1.summary()
Out[37]:
In [38]:
fitted1.rsquared
Out[38]:
- How are Sem2_Math & Final score related? As Sem2_Math score increases, what happens to Final score?
As Sem2_Math score increases Final score decreases
In [39]:
#Remove "Sem1_Math" variable from the model and rebuild the model
from sklearn.linear_model import LinearRegression
lr2 = LinearRegression()
lr2.fit(final_exam[["Sem1_Science"]+["Sem2_Science"]+["Sem2_Math"]], final_exam[["Final_exam_marks"]])
predictions2 = lr2.predict(final_exam[["Sem1_Science"]+["Sem2_Science"]+["Sem2_Math"]])
import statsmodels.formula.api as sm
model2 = sm.ols(formula='Final_exam_marks ~ Sem1_Science+Sem2_Science+Sem2_Math', data=final_exam)
fitted2 = model2.fit()
fitted2.summary()
Out[39]:
- Is there any change in R square or Adj R square
Model | R2 |
---|
AdjR2 |
---|
model1 | 0.990 | 0.987 | |
model2 | 0.981 | 0.978 |
- How are Sem2_Math & Final score related now? As Sem2_Math score increases, what happens to Final score?
As Sem2_Math score increases Final score also increases.
In [40]:
#Draw a scatter plot between Sem1_Math & Sem2_Mat
import matplotlib.pyplot as plt
%matplotlib inline
plt.scatter(final_exam.Sem1_Math,final_exam.Sem2_Math)
Out[40]:
In [41]:
#Find the correlation between Sem1_Math & Sem2_Math
np.corrcoef(final_exam.Sem1_Math,final_exam.Sem2_Math)
Out[41]:
Link to the next post : https://statinfer.com/204-1-9-issue-of-multicollinearity-in-python/