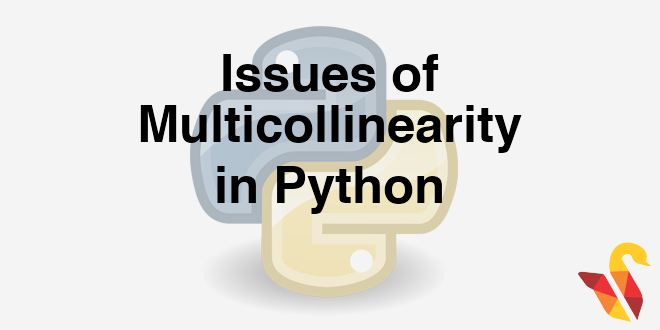
In previous post of this series we looked into the issues with Multiple Regression models. In this part we will understand what Multicollinearity is and how it’s bad for the model.
We will also go through the measures of Multicollinearity and how to deal with it.
Multicollinearity
- Multiple regression is wonderful – It allows you to consider the effect of multiple variables simultaneously.
- Multiple regression is extremely unpleasant -Because it allows you to consider the effect of multiple variables simultaneously.
- The relationships between the explanatory variables are the key to understanding multiple regression.
- Multicollinearity (or inter correlation) exists when at least some of the predictor variables are correlated among themselves.
- The parameter estimates will have inflated variance in presence of multicollineraity.
- Sometimes the signs of the parameter estimates tend to change.
- If the relation between the independent variables grows really strong then the variance of parameter estimates tends to be infinity – Can you prove it?
Multicollinearity Detection
Y=β0+β1X1+β2X2+β3X3+β4X4
- Build a model X1 vs X2 X3 X4 find R^2, say R1
- Build a model X2 vs X1 X3 X4 find R^2, say R2
- Build a model X3 vs X1 X2 X4 find R^2, say R3
- Build a model X4 vs X1 X2 X3 find R^2, say R4
- For example if R3 is 95% then we don’t really need X3 in the model.
- Since it can be explained as liner combination of other three.
- For each variable we find individual R2.
- 1/(1−R^2) is called VIF.
- VIF option in SAS automatically calculates VIF values for each of the predictor variables
R2 | 40% | 50% | 60% | 70% | 75% | 80% | 90% |
---|---|---|---|---|---|---|---|
VIF | 1.67 | 2.00 | 2.50 | 3.33 | 4.00 | 5.00 | 10.00 |
Practice : Multicollinearity
- Identify the Multicollinearity in the Final Exam Score model.
- Drop the variable one by one to reduce the multicollinearity.
In [42]:
from sklearn.linear_model import LinearRegression
lr1 = LinearRegression()
lr1.fit(final_exam[["Sem1_Science"]+["Sem2_Science"]+["Sem1_Math"]+["Sem2_Math"]], final_exam[["Final_exam_marks"]])
predictions1 = lr1.predict(final_exam[["Sem1_Science"]+["Sem2_Science"]+["Sem1_Math"]+["Sem2_Math"]])
In [44]:
import statsmodels.formula.api as sm
model1 = sm.ols(formula='Final_exam_marks ~ Sem1_Science+Sem2_Science+Sem1_Math+Sem2_Math', data=final_exam)
fitted1 = model1.fit()
fitted1.summary()
Out[44]:
In [45]:
fitted1.summary2()
Out[45]:
In [48]:
There is no function for calculating VIF values.
None of the pre-built libraries have this VIF calculation function
We may have to write our own function to calculate VIF values for each variable
#Code for VIF Calculation
#Writing a function to calculate the VIF values
def vif_cal(input_data, dependent_col):
x_vars=input_data.drop([dependent_col], axis=1)
xvar_names=x_vars.columns
for i in range(0,xvar_names.shape[0]):
y=x_vars[xvar_names[i]]
x=x_vars[xvar_names.drop(xvar_names[i])]
rsq=sm.ols(formula="y~x", data=x_vars).fit().rsquared
vif=round(1/(1-rsq),2)
print (xvar_names[i], " VIF = " , vif)
In [49]:
#Calculating VIF values using that function
vif_cal(input_data=final_exam, dependent_col="Final_exam_marks")
In [51]:
import statsmodels.formula.api as sm
model2 = sm.ols(formula='Final_exam_marks ~ Sem1_Science+Sem2_Science+Sem2_Math', data=final_exam)
fitted2 = model2.fit()
fitted2.summary()
Out[51]:
In [52]:
vif_cal(input_data=final_exam.drop(["Sem1_Math"], axis=1), dependent_col="Final_exam_marks")
In [53]:
vif_cal(input_data=final_exam.drop(["Sem1_Math","Sem1_Science"], axis=1), dependent_col="Final_exam_marks")