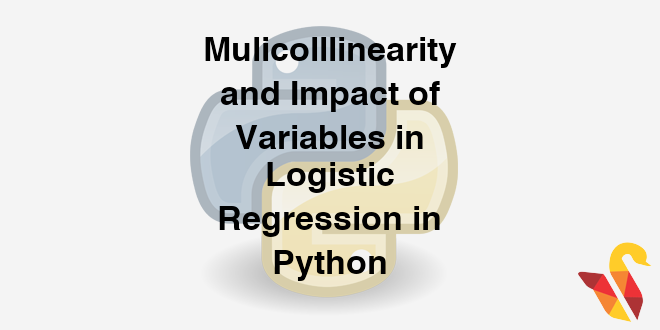
Link to the previous post : https://statinfer.com/204-2-4-goodness-of-fit-for-logistic-regression/
Previous post was about goodness of fit, we covered Confusion matrix and will cover the rest in next posts too.
But, first let’s deal with a common issue with modeling:
Multicollinearity
- The relation between X and Y is non linear, we used logistic regression.
- The multicollinearity is an issue related to predictor variables.
- Multicollinearity need to be fixed in logistic regression as well.
- Otherwise the individual coefficients of the predictors will be effected by the inter-dependency.
- The process of identification is same as linear regression.
Practice : Multicollinearity
- Is there any multicollinearity in fiber bits model?
- Identify and remove multicollinearity from the model
In [27]:
def vif_cal(input_data, dependent_col):
x_vars=input_data.drop([dependent_col], axis=1)
xvar_names=x_vars.columns
for i in range(0,xvar_names.shape[0]):
y=x_vars[xvar_names[i]]
x=x_vars[xvar_names.drop(xvar_names[i])]
rsq=sm.ols(formula="y~x", data=x_vars).fit().rsquared
vif=round(1/(1-rsq),2)
print (xvar_names[i], " VIF = " , vif)
In [28]:
#Calculating VIF values using that function
vif_cal(input_data=Fiber, dependent_col="active_cust")
Individual Impact of Variables
- Out of these predictor variables, what are the important variables?
- If we have to choose the top 5 variables what are they?
- While selecting the model, we may want to drop few less impacting variables.
- How to rank the predictor variables in the order of their importance?
- We can simply look at the z values of the each variable. Look at their absolute values.
- Or calculate the Wald chi-square, which is nearly equal to square of the z-score.
- Wald Chi-Square value helps in ranking the variables.
Practice : Individual Impact of Variables
- Identify top impacting and least impacting variables in fiber bits models.
- Find the variable importance and order them based on their impact.
In [29]:
result1.summary()
Out[29]:
Top impacting variables are – relocated & Speed_test_result.
Least impacting variables are – monthly_bill & income.
The next post is about model selection logistic regression.
Link to the next post : https://statinfer.com/204-2-6-model-selection-logistic-regression/