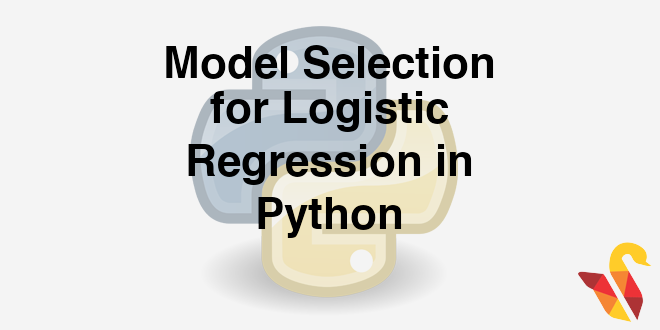
Link to the previous post : https://statinfer.com/204-2-5-multicollinearity-and-individual-impact-of-variables-in-logistic-regression/
We left some part of the post regarding goodness of fitness behind. We will cover them in this post and see if we can improve our model based on AIC and BIC.
We will also cover various methods used for model selection in a series dedicated to it.
How to improve model
- By adding more independent variables?
- By deriving new variables from available set?
- By transforming variables ?
- By collecting more data?
- How do we choose best model from the list of fitted models with different parameters
AIC and BIC
- AIC and BIC values are like adjusted R-squared values in linear regression.
- Stand-alone model AIC has no real use, but if we are choosing between the models AIC really helps.
- Given a collection of models for the data, AIC estimates the quality of each model, relative to each of the other models.
- If we are choosing between two models, a model with less AIC is preferred.
- AIC is an estimate of the information lost when a given model is used to represent the process that generates the data.
- AIC= -2ln(L)+ 2k
- L be the maximum value of the likelihood function for the model.
- k is the number of independent variables.
- BIC is a substitute to AIC with a slightly different formula. We will follow either AIC or BIC throughout our analysis.
Practice : Logistic Regression Model Selection
- Find AIC and BIC values for the first fiber bits model(m1)
- What are the top-2 impacting variables in fiber bits model?
- What are the least impacting variables in fiber bits model?
- Can we drop any of these variables and build a new model(m2)
- Can we add any new interaction and polynomial variables to increase the accuracy of the model?(m3)
- We have three models, what the best accuracy that you can expect on this data?
In [30]:
#Find AIC and BIC values for the first fiber bits model(m2)
m1=sm.Logit(Fiber['active_cust'],Fiber[["income"]+['months_on_network']+['Num_complaints']+['number_plan_changes']+['relocated']+['monthly_bill']+['technical_issues_per_month']+['Speed_test_result']])
m1
m1.fit()
m1.fit().summary2()
Out[30]:
- What are the top-2 impacting variables in fiber bits model?
- What are the least impacting variables in fiber bits model?
In [31]:
m1.fit().summary()
Out[31]:
- Can we drop any of these variables and build a new model(m2)
In [32]:
#Income and Monthly Bill Dropped because those are the least impacting variables
m2=sm.Logit(Fiber['active_cust'],Fiber[['months_on_network']+['Num_complaints']+['number_plan_changes']+['relocated']+['technical_issues_per_month']+['Speed_test_result']])
m2
m2.fit()
m2.fit().summary()
m2.fit().summary2()
Out[32]:
Conclusion: Logistic Regression
- Logistic Regression is the base of all classification algorithms.
- A good understanding on logistic regression and goodness of fit measures will really help in understanding complex machine learning algorithms like neural networks and SVMs.
- One has to be careful while selecting the model, all the goodness of fit measures are calculated on training data. We may have to do cross validation to get an idea on the test error.