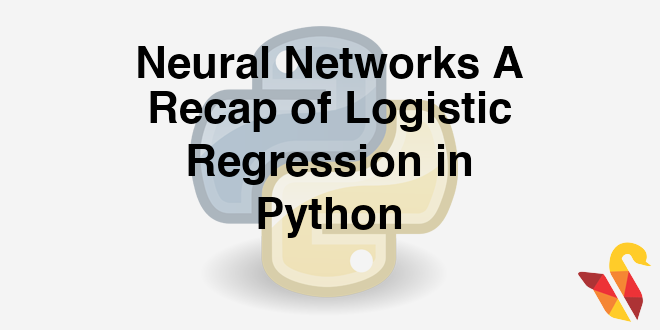
Welcome to this Blog series on Neural Networks. In this series 204.5 we will go from basics of neural networks to build a neural network model that recognizes digit images and reads them correctly.
In this post, we will just revise our understanding of how logistic regression works, which can be considered a building block for a neural network.
Recap of Logistic Regression
- Categorical output YES/NO type.
- Using the predictor variables to predict the categorical output.
Practice : Logistic Regression
- Dataset: Emp_Productivity/Emp_Productivity.csv
- Filter the data and take a subset from above dataset . Filter condition is Sample_Set<3.
- Draw a scatter plot that shows Age on X axis and Experience on Y-axis. Try to distinguish, the two classes with colors or shapes (visualizing the classes).
- Build a logistic regression model to predict Productivity using age and experience.
- Finally, draw the decision boundary for this logistic regression model.
- Create, the confusion matrix.
- Calculate, the accuracy and error rates.
Solution
In [1]:
import pandas as pd
Emp_Productivity_raw = pd.read_csv("datasets\\Emp_Productivity\\Emp_Productivity.csv")
Emp_Productivity_raw.head(10)
Out[1]:
In [2]:
#Filter the data and take a subset from above dataset . Filter condition is Sample_Set<3
Emp_Productivity1=Emp_Productivity_raw[Emp_Productivity_raw.Sample_Set<3]
Emp_Productivity1.shape
Out[2]:
In [3]:
#frequency table of Productivity variable
Emp_Productivity1.Productivity.value_counts()
Out[3]:
In [4]:
####The clasification graph
#Draw a scatter plot that shows Age on X axis and Experience on Y-axis. Try to distinguish the two classes with colors or shapes.
import matplotlib.pyplot as plt
%matplotlib inline
fig = plt.figure()
ax1 = fig.add_subplot(111)
ax1.scatter(Emp_Productivity1.Age[Emp_Productivity1.Productivity==0],Emp_Productivity1.Experience[Emp_Productivity1.Productivity==0], s=10, c='b', marker="o", label='Productivity 0')
ax1.scatter(Emp_Productivity1.Age[Emp_Productivity1.Productivity==1],Emp_Productivity1.Experience[Emp_Productivity1.Productivity==1], s=10, c='r', marker="+", label='Productivity 1')
plt.legend(loc='upper left');
plt.show()
In [5]:
#predict Productivity using age and experience
###Logistic Regerssion model1
import statsmodels.formula.api as sm
model1 = sm.logit(formula='Productivity ~ Age+Experience', data=Emp_Productivity1)
fitted1 = model1.fit()
fitted1.summary()
Out[5]:
In [6]:
#coefficients
coef=fitted1.normalized_cov_params
print(coef)
In [7]:
# getting slope and intercept of the line
slope1=coef.Intercept[1]/(-coef.Intercept[2])
intercept1=coef.Intercept[0]/(-coef.Intercept[2])
slope1
intercept1
Out[7]:
In [8]:
#Finally draw the decision boundary for this logistic regression model
import matplotlib.pyplot as plt
fig = plt.figure()
ax1 = fig.add_subplot(111)
ax1.scatter(Emp_Productivity1.Age[Emp_Productivity1.Productivity==0],Emp_Productivity1.Experience[Emp_Productivity1.Productivity==0], s=10, c='b', marker="o", label='Productivity 0')
ax1.scatter(Emp_Productivity1.Age[Emp_Productivity1.Productivity==1],Emp_Productivity1.Experience[Emp_Productivity1.Productivity==1], s=10, c='r', marker="+", label='Productivity 1')
plt.legend(loc='upper left');
x_min, x_max = ax1.get_xlim()
ax1.plot([0, x_max], [intercept1, x_max*slope1+intercept1])
ax1.set_xlim([15,35])
ax1.set_ylim([0,10])
plt.show()
- Accuracy of the model
In [9]:
#Predicting classes
predicted_values=fitted1.predict(Emp_Productivity1[["Age"]+["Experience"]])
predicted_values[1:10]
threshold=0.5
threshold
import numpy as np
predicted_class=np.zeros(predicted_values.shape)
predicted_class[predicted_values>threshold]=1
predicted_class
Out[9]:
In [10]:
#Confusion Matrix, Accuracy and Error
from sklearn.metrics import confusion_matrix as cm
ConfusionMatrix = cm(Emp_Productivity1[['Productivity']],predicted_class)
print('Confusion Matrix :', ConfusionMatrix)
accuracy=(ConfusionMatrix[0,0]+ConfusionMatrix[1,1])/sum(sum(ConfusionMatrix))
print('Accuracy : ',accuracy)
error=1-accuracy
print('Error: ',error)