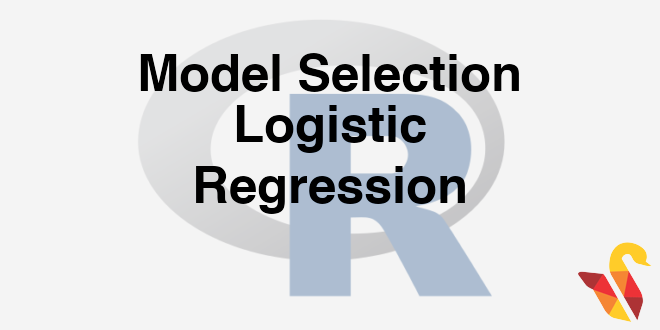
LAB-Logistic Regression Model Selection
In previous section, we studied about Multi-collinearity and Individual Impact Of Variables in Logistic Regression
-
- What are the top-2 impacting variables in fiber bits model?
-
- What are the least impacting variables in fiber bits model?
-
- Can we drop any of these variables?
-
- Can we derive any new variables to increase the accuracy of the model?
-
- What is the final model? What the best accuracy that you can expect on this data?
Solution
-
- What are the top-2 impacting variables in fiber bits model?
Speed_test_result and relocation status are the top two important variables
-
- What are the least impacting variables in fiber bits model?
monthly_bill and income are the least impacting variables
-
- Can we drop any of these variables?
We can drop monthly_bill and income, they have the least impact when compared to other predictors. But we need to see the accuracy and AIC then take the final decision.
AIC & Accuracy of Model1
threshold=0.5
predicted_values<-ifelse(predict(Fiberbits_model_1,type="response")>threshold,1,0)
actual_values<-Fiberbits_model_1$y
conf_matrix<-table(predicted_values,actual_values)
conf_matrix
## actual_values
## predicted_values 0 1
## 0 29492 10847
## 1 12649 47012
accuracy1<-(conf_matrix[1,1]+conf_matrix[2,2])/(sum(conf_matrix))
accuracy1
## [1] 0.76504
AIC(Fiberbits_model_1)
## [1] 98377.36
AIC & Accuracy of Model1 without monthly_bill
Fiberbits_model_11<-glm(active_cust~.-monthly_bill,family=binomial(),data=Fiberbits)
## Warning: glm.fit: fitted probabilities numerically 0 or 1 occurred
threshold=0.5
predicted_values<-ifelse(predict(Fiberbits_model_11,type="response")>threshold,1,0)
actual_values<-Fiberbits_model_11$y
conf_matrix<-table(predicted_values,actual_values)
accuracy11<-(conf_matrix[1,1]+conf_matrix[2,2])/(sum(conf_matrix))
accuracy11
## [1] 0.76337
AIC(Fiberbits_model_11)
## [1] 98580.54
AIC & Accuracy of Model1 without income
Fiberbits_model_2<-glm(active_cust~.-income,family=binomial(),data=Fiberbits)
## Warning: glm.fit: fitted probabilities numerically 0 or 1 occurred
threshold=0.5
predicted_values<-ifelse(predict(Fiberbits_model_2,type="response")>threshold,1,0)
actual_values<-Fiberbits_model_2$y
conf_matrix<-table(predicted_values,actual_values)
accuracy2<-(conf_matrix[1,1]+conf_matrix[2,2])/(sum(conf_matrix))
accuracy2
## [1] 0.76695
AIC(Fiberbits_model_2)
## [1] 99076.27
Deciding which Variable to Drop
Model | All Variables | Without monthly_bill | Without income |
---|---|---|---|
AIC | 98377.36 | 98580.54 | 99076.27 |
Accuracy | 0.76504 | 0.76337 | 0.76695 |
Dropping Income has not reduced the accuracy. AIC(Loss of information) also shows no big change.
Output of Model2
summary(Fiberbits_model_2)
##
## Call:
## glm(formula = active_cust ~ . - income, family = binomial(),
## data = Fiberbits)
##
## Deviance Residuals:
## Min 1Q Median 3Q Max
## -8.4904 -0.8901 0.4175 0.7675 3.1083
##
## Coefficients:
## Estimate Std. Error z value Pr(>|z|)
## (Intercept) -1.309e+01 2.100e-01 -62.34 <2e-16 ***
## months_on_network 1.004e-02 4.644e-04 21.62 <2e-16 ***
## Num_complaints -7.071e-01 2.990e-02 -23.65 <2e-16 ***
## number_plan_changes -2.016e-01 7.571e-03 -26.63 <2e-16 ***
## relocated -3.133e+00 3.933e-02 -79.66 <2e-16 ***
## monthly_bill -2.253e-03 1.566e-04 -14.39 <2e-16 ***
## technical_issues_per_month -3.970e-01 7.159e-03 -55.45 <2e-16 ***
## Speed_test_result 2.198e-01 2.334e-03 94.16 <2e-16 ***
## ---
## Signif. codes: 0 '***' 0.001 '**' 0.01 '*' 0.05 '.' 0.1 ' ' 1
##
## (Dispersion parameter for binomial family taken to be 1)
##
## Null deviance: 136149 on 99999 degrees of freedom
## Residual deviance: 99060 on 99992 degrees of freedom
## AIC: 99076
##
## Number of Fisher Scoring iterations: 7
-
- Can we derive any new variables to increase the accuracy of the model?
Fiberbits_model_3<-glm(active_cust~ income
+months_on_network
+Num_complaints
+number_plan_changes
+relocated
+monthly_bill
+technical_issues_per_month
+technical_issues_per_month*number_plan_changes
+Speed_test_result+I(Speed_test_result^2),
family=binomial(),data=Fiberbits)
## Warning: glm.fit: fitted probabilities numerically 0 or 1 occurred
summary(Fiberbits_model_3)
##
## Call:
## glm(formula = active_cust ~ income + months_on_network + Num_complaints +
## number_plan_changes + relocated + monthly_bill + technical_issues_per_month +
## technical_issues_per_month * number_plan_changes + Speed_test_result +
## I(Speed_test_result^2), family = binomial(), data = Fiberbits)
##
## Deviance Residuals:
## Min 1Q Median 3Q Max
## -4.6112 -0.8478 0.3780 0.7401 2.9909
##
## Coefficients:
## Estimate Std. Error
## (Intercept) -2.501e+01 3.647e-01
## income 1.831e-03 8.386e-05
## months_on_network 2.905e-02 1.011e-03
## Num_complaints -6.972e-01 3.030e-02
## number_plan_changes -4.404e-01 2.199e-02
## relocated -3.253e+00 3.997e-02
## monthly_bill -2.295e-03 1.588e-04
## technical_issues_per_month -4.670e-01 9.694e-03
## Speed_test_result 3.910e-01 4.260e-03
## I(Speed_test_result^2) -9.438e-04 1.272e-05
## number_plan_changes:technical_issues_per_month 7.481e-02 6.164e-03
## z value Pr(>|z|)
## (Intercept) -68.56 <2e-16 ***
## income 21.83 <2e-16 ***
## months_on_network 28.73 <2e-16 ***
## Num_complaints -23.00 <2e-16 ***
## number_plan_changes -20.03 <2e-16 ***
## relocated -81.39 <2e-16 ***
## monthly_bill -14.46 <2e-16 ***
## technical_issues_per_month -48.17 <2e-16 ***
## Speed_test_result 91.79 <2e-16 ***
## I(Speed_test_result^2) -74.20 <2e-16 ***
## number_plan_changes:technical_issues_per_month 12.14 <2e-16 ***
## ---
## Signif. codes: 0 '***' 0.001 '**' 0.01 '*' 0.05 '.' 0.1 ' ' 1
##
## (Dispersion parameter for binomial family taken to be 1)
##
## Null deviance: 136149 on 99999 degrees of freedom
## Residual deviance: 97105 on 99989 degrees of freedom
## AIC: 97127
##
## Number of Fisher Scoring iterations: 7
AIC & Accuracy of Model 3
threshold=0.5
predicted_values<-ifelse(predict(Fiberbits_model_3,type="response")>threshold,1,0)
actual_values<-Fiberbits_model_3$y
conf_matrix<-table(predicted_values,actual_values)
accuracy3<-(conf_matrix[1,1]+conf_matrix[2,2])/(sum(conf_matrix))
accuracy3
## [1] 0.76061
AIC(Fiberbits_model_3)
## [1] 97127.17
-
- What is the final model? What the best accuracy that you can expect on this data?
AIC(Fiberbits_model_1,Fiberbits_model_2,Fiberbits_model_3)
## df AIC
## Fiberbits_model_1 9 98377.36
## Fiberbits_model_2 8 99076.27
## Fiberbits_model_3 11 97127.17
accuracy1
## [1] 0.76504
accuracy2
## [1] 0.76695
accuracy3
## [1] 0.76061
Conclusion: Logistic Regression
- Logistic Regression is the base of all classification algorithms.
- A good understanding on logistic regression and goodness of fit measures will really help in understanding complex machine learning algorithms like neural networks and SVMs.
- One has to be careful while selecting the model, all the goodness of fit measures are calculated on training data.
- We may have to do cross validation to get an idea on the test error.
In next section we will be studying about Decision Trees in r : Segmentation