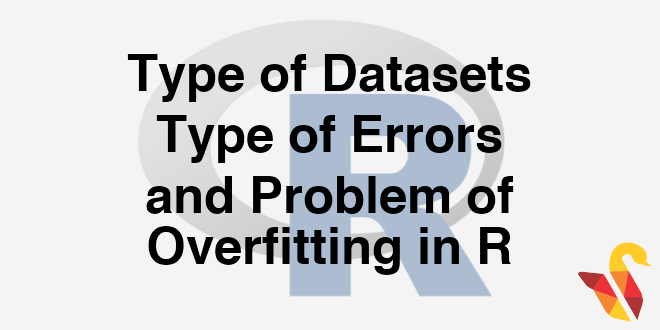
The Problem of Over Fitting
In previous section, we studied about What is a Best Model?
- In search of the best model on the given data we add many predictors, polynomial terms, Interaction terms, variable transformations, derived variables, indicator/dummy variables etc.,
- Most of the times we succeed in reducing the error. What error is this?
- So by complicating the model we fit the best model for the training data.
- Sometimes the error on the training data can reduce to near zero
- But the same best model on training data fails miserably on test data.
- Imagine building multiple models with small changes in training data. The resultant set of models will have huge variance in their parameter estimates.
- The model is made really complicated, that it is very sensitive to minimal changes
- By complicating the model the variance of the parameters estimates inflates
- Model tries to fit the irrelevant characteristics in the data
- Over fitting
- The model is super good on training data but not so good on test data
- We fit the model for the noise in the data
- Less training error, high testing error
- The model is over complicated with too many predictors
- Model need to be simplified
- A model with lot of variance
LAB: Model with huge Variance
- Data: Fiberbits/Fiberbits.csv
- Take initial 90% of the data. Consider it as training data. Keep the final 10% of the records for validation.
- Build the best model(5% error) model on training data.
- Use the validation data to verify the error rate. Is the error rate on the training data and validation data same?
Solution
fiber_bits_train<-Fiberbits[1:90000,]
fiber_bits_validation<-Fiberbits[90001:100000,]
Model on training data
Fiber_bits_tree3<-rpart(active_cust~., method="class", control=rpart.control(minsplit=5, cp=0.000001), data=fiber_bits_train)
Fbits_pred3<-predict(Fiber_bits_tree3, type="class")
conf_matrix3<-table(Fbits_pred3,fiber_bits_train$active_cust)
accuracy3<-(conf_matrix3[1,1]+conf_matrix3[2,2])/(sum(conf_matrix3))
accuracy3
## [1] 0.9524889
Validation Accuracy
fiber_bits_validation$pred <- predict(Fiber_bits_tree3, fiber_bits_validation,type="class")
conf_matrix_val<-table(fiber_bits_validation$pred,fiber_bits_validation$active_cust)
accuracy_val<-(conf_matrix_val[1,1]+conf_matrix_val[2,2])/(sum(conf_matrix_val))
accuracy_val
## [1] 0.7116
Error rate on validation data is more than the training data error.
The Problem of Under-fitting
- Simple models are better. Its true but is that always true? May not be always true.
- We might have given it up too early. Did we really capture all the information?
- Did we do enough research and future reengineering to fit the best model? Is it the best model that can be fit on this data?
- By being over cautious about variance in the parameters, we might miss out on some patterns in the data.
- Model need to be complicated enough to capture all the information present.
- If the training error itself is high, how can we be so sure about the model performance on unknown data?
- Most of the accuracy and error measuring statistics give us a clear idea on training error, this is one advantage of under fitting, we can identify it confidently.
- Under fitting
- A model that is too simple
- A mode with a scope for improvement
- A model with lot of bias
LAB: Model with huge Bias
- Lets simplify the model.
- Take the high variance model and prune it.
- Make it as simple as possible.
- Find the training error and validation error.
Solution
- Simple Model
Fiber_bits_tree4<-rpart(active_cust~., method="class", control=rpart.control(minsplit=30, cp=0.25), data=fiber_bits_train)
prp(Fiber_bits_tree4)
Fbits_pred4<-predict(Fiber_bits_tree4, type="class")
conf_matrix4<-table(Fbits_pred4,fiber_bits_train$active_cust)
conf_matrix4
##
## Fbits_pred4 0 1
## 0 11209 921
## 1 25004 52866
accuracy4<-(conf_matrix4[1,1]+conf_matrix4[2,2])/(sum(conf_matrix4))
accuracy4
## [1] 0.7119444
- Validation accuracy
fiber_bits_validation$pred1 <- predict(Fiber_bits_tree4, fiber_bits_validation,type="class")
conf_matrix_val1<-table(fiber_bits_validation$pred1,fiber_bits_validation$active_cust)
accuracy_val1<-(conf_matrix_val1[1,1]+conf_matrix_val1[2,2])/(sum(conf_matrix_val1))
accuracy_val1
## [1] 0.4224
The next post is about Model Bias Variance Tradeoff.